In airport operations, technologies like LiDAR, 3D stereo vision sensors, and WiFi sensors have long been standard for improving efficiency and safety. However, the rise of AI and machine learning is changing the game.
With companies like Tesla moving away from LiDAR in favor of AI for autonomous driving, airport tech leaders are now facing a big decision. How should they choose the right technology for their needs in an industry as complex as aviation? This shift to AI, especially in computer vision, could be a game-changer for airports.
The “necessary evil”
Airports have historically turned to legacy sensors for supporting various airport initiatives. These were used to streamline various airport operations, provide a smoother travel experience, ensure the safety of passengers and staff, and make data-driven decisions.
However, deploying these legacy sensors has consistently come with its own set of significant challenges. These decreased their usefulness in different airport initiatives, regardless of the sensor type.
The dilemma: cost vs accuracy
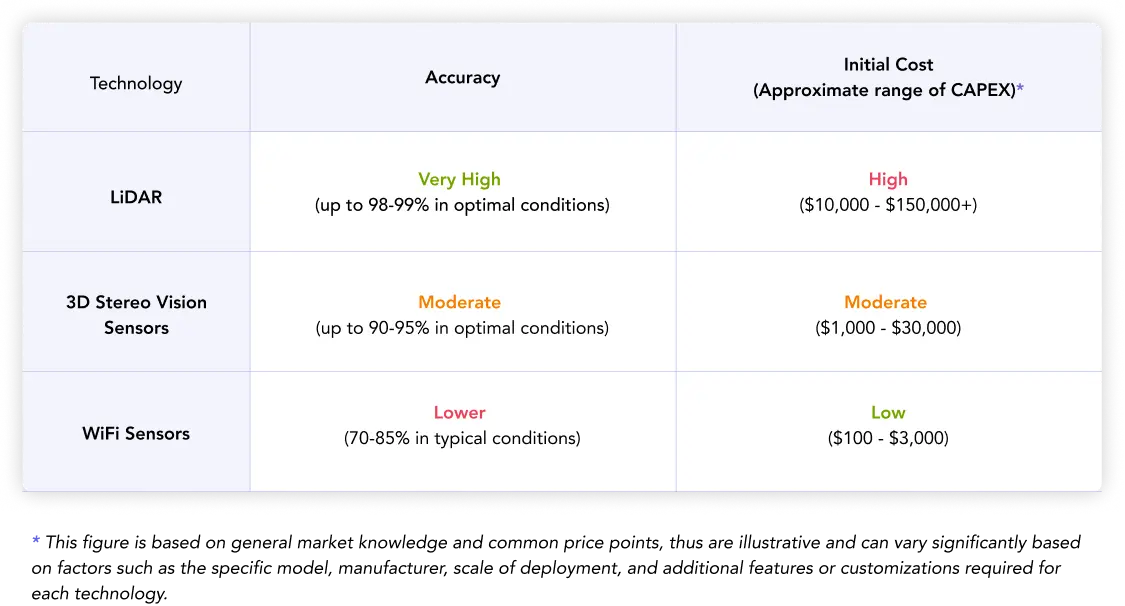
Opting for lower-cost options often meant sacrificing accuracy. Furthermore, integrating these sensors into existing airport setups often resulted in underperformance. This typically necessitated additional installations, further escalating costs.
Traditional LiDAR systems, while accurate in obstacle detection, fail in environments with glass and mirrors like airport terminals. The glares and reflections interfere with their laser pulses, hindering accurate 3D mapping. Similarly, 3D stereo vision sensors face limitations when installed on low ceilings, requiring more units and increased costs to achieve full coverage.
Low service standard
Legacy sensors, having been around for a long time and often installed years ago, didn't need to offer superior service and user experience. Maintenance typically required specialized technicians, leading to delays that were dictated by their availability rather than the airport's immediate needs.
These older systems also struggle to integrate seamlessly with modern data platforms. They often require extensive data cleansing and custom data pipeline development for airports to utilize the data effectively in their BI platforms.
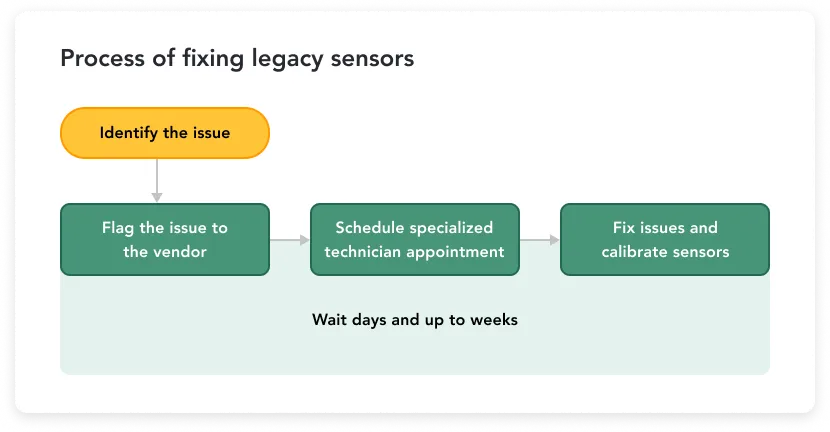
New sensors for new initiatives
In the pre-AI era, the capabilities of software and hardware relied heavily on developers, which meant sensors, regardless of the technologies used, were often developed and calibrated for single use cases. This forced airports to procure multiple solutions from various vendors for a holistic operational view.
The end result was a fragmented user experience, with airport staff having to navigate multiple software interfaces and data teams investing significant effort to create centralized BI dashboards and reports.
Why legacy sensors are doomed
Tesla’s decision to remove LiDAR from its autonomous driving systems points to a broader industry trend. Computer vision, paired with AI, presents itself as a more cost-effective, accurate, and faster solution for modern technology needs.
Computer vision gets AI makeover
Previously, computer vision worked similarly to legacy sensors. It relied on developers to define specific rules for identifying people or objects, a process known as “supervised learning.” This approach was resource-intensive, costly, and limited in scope, struggling with complexity and demanding perfect conditions.
However, the introduction of deep learning AI algorithms has charted a new era for airport technologies. Employing neural networks like how the human brain functions, has enabled computer vision systems to learn how to identify complex patterns and objects on their own.
It drastically improved accuracy even in “imperfect conditions” with occlusions from long distances, glares from light, low light environments, removing the need for LiDAR - the most expensive and accurate of them all.
By moving from intense manual work of humans to full automation, computer vision started improving its performance continuously, allowing lower cost and rapid deployment to any environment.
Supports dynamic changes at airports
This means more than just getting accuracy in imperfect conditions, especially at airports. With so many people and processes at play, the operations of the terminal also change frequently based on the number of passengers at a certain checkpoint. This was one of the biggest challenge for Toronto Pearson in improving passenger flow.
Legacy sensors, which relied on human developers to configure new operational processes every time they changed, often failed to capture what’s going on, from when the change happened to when the vendor support team updates the system.
With AI processing, learning, and improving data in real-time, computer vision supports these changes almost instantly. For example, AI automatically captures the full queue, even when it overflowed from the typical queue area.
When activating an alternate checkpoint, the airport operations team can simply click a button to notify AI of the change, and within seconds AI will start collecting the data in the new context. This immediate adaptability not only enhances operational accuracy but also boosts efficiency, a key consideration in busy airport environments.
Is it the end of LiDAR and sensors?
Vision AI (Computer vision enhanced with AI), uses existing security cameras in airport terminals. Airports no longer have to waste significant CAPEX these legacy sensors often required. What’s more, it improves performance continuously, when all other legacy sensors stay the same.
It’s no longer choosing the “necessary evil” between cost and accuracy, nor dealing with vendors' sub-par support and lagging timelines. With the development of technology, airports now have a cheaper, better, and faster option.

AI is also making the dreams of visionary airport IT leaders come true - a single AI solution for all their technology needs, not 5 solutions for 5 use cases. When there’s a new initiative, leaders simply train the AI on the new task and environment, rather than procuring a new point solution.
Procuring new solutions and time-to-value now only take days rather than months to years. As AI continues to evolve, it's not just replacing old technology; it's reshaping the entire landscape of airport operations, making them smarter, more efficient, and future-ready.
Renew or transition?
Airport IT leaders face a tough choice: update their tech to AI or keep the old systems going. Transitioning isn't just a tech swap; it's a big deal in a busy place like an airport where everything's connected.
Change can be tricky when you're dealing with so many moving parts. AI's getting better and doing things old sensors can't, but how can airport leaders make this switch without causing a stir in their day-to-day operations? Is there a smart, doable way to bring in AI?
Where LiDAR is still relevant
While LiDAR remains relevant in scenarios requiring precise depth and spatial measurements, such as runway and taxiway inspections, recent developments suggest a shift.
Drones and AI in computer vision are increasingly being used for these purposes, as highlighted by Pearson Airport's recent runway inspection trials. Even terrain and airspace mapping are seeing promising developments using computer vision and deep learning AI.
It leaves airport IT leaders with a decision: stick with a reliable but aging technology, or adapt to the evolving AI era? Weighing LiDAR's proven reliability against the versatility and potential of AI that can outperform legacy technology is key to making an informed choice.
Start small and quick
Transitioning or change management, if done right, can be straightforward. Instead of a complete overhaul, start small and scale up. This is particularly true for AI, which can be deployed within days and adapts to unique environments.
While embarking on this transition, it's crucial for airports to keep the end goal in mind: managing end-to-end service levels across stakeholders, enhancing passenger experience, or improving metrics like Airport Service Quality (ASQ) and end-to-end Level of Service (LoS).
Begin with areas where you have end-of-life sensors or where gaps in operational knowledge exist. This approach allows airports to leverage existing infrastructure while effectively integrating AI. With an end-goal, airports can align each step with broader strategic objectives like improving overall passenger experience and operational efficiency.
For instance, deploying AI to enhance passenger flow analytics at a single checkpoint, where currently missing, can provide immediate benefits. This initial success can set a positive tone for broader AI adoption, demonstrating its potential impact on service levels and passenger satisfaction.
Exploring AI as a replacement for end-of-life sensors can gradually reduce dependency on multiple vendors. This not only streamlines operations and vendor management but also ensures a quicker time-to-value.
Each step towards a more integrated, AI-driven future in airport management should contribute to overarching goals like maintaining high service standards and optimizing the passenger journey.
Conclusion
The shift from traditional sensors like LiDAR to AI-driven technologies such as computer vision signifies a major step forward in airport technology. Building on existing change management experiences from past technological adaptations, airports can now adopt more cost-effective and advanced AI solutions.
This transition not only promises enhanced operational efficiency and flexibility but also positions airports to be more adaptable and efficient in the future. Embracing AI-driven technology is an opportunity for airports to refine their operations with smarter, more effective systems.
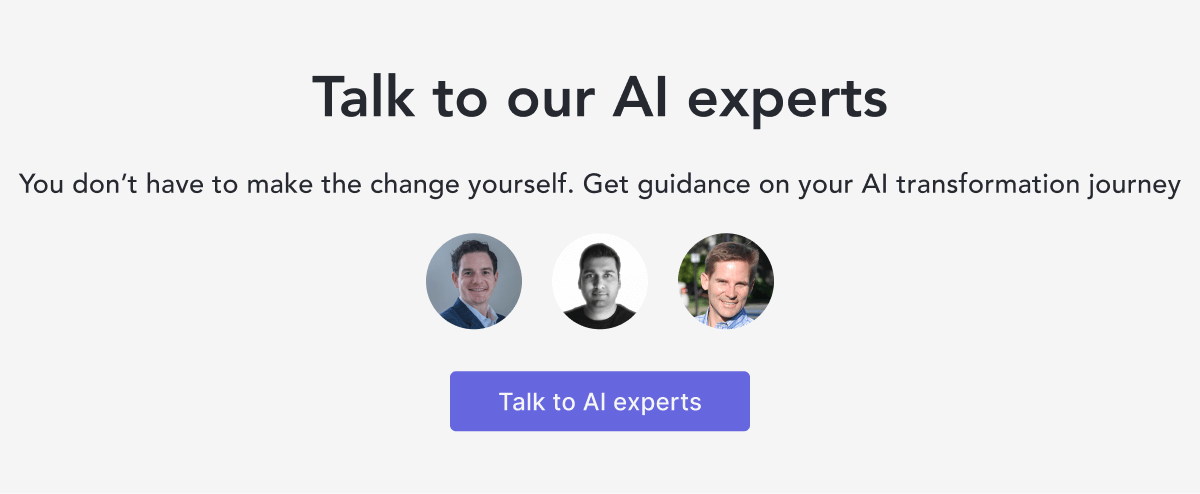